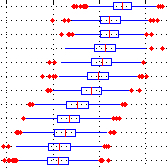
Papers
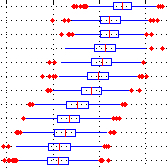
In this paper, we provide a framework for the evaluation of visual separation measures. We used the framework to evaluate 15 existing state-of-the-art measures. The best one has been found to be the so-called DSC measure from Sips et al. (EuroVis 2009).
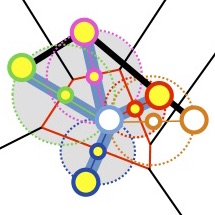
In this paper, we propose a set of 2002 new visual separation measures. The measures are based on a systematic combination of 17 neighborhood graphs and 14 class purity functions, with different parameterizations. We found that 58.4% of these measures performed better than the best state-of-the-art measure DSC. We also identify the best among the 2002, and discuss alternativ choices.
Previous Work
Our work on visual separation measures builds up on previous work together with Tamara Munzner, Melanie Tory, and Andrada Tatu. Specifically, we build upon findings and data from two papers:
A Taxonomy of Visual Cluster Separation Factors
Michael Sedlmair, Andrada Tatu, Tamara Munzner, and Melanie Tory
Computer Graphics Forum (Proc. EuroVis), 31(3), 1335-1344, 2012.
→ External paper page
Empirical Guidance on Scatterplot and Dimension Reduction Technique Choices
Michael Sedlmair, Tamara Munzner, and Melanie Tory
IEEE Trans. Visualization and Computer Graphics (Proc. InfoVist), 19(12): 2634-2643, 2013.
→ External paper page